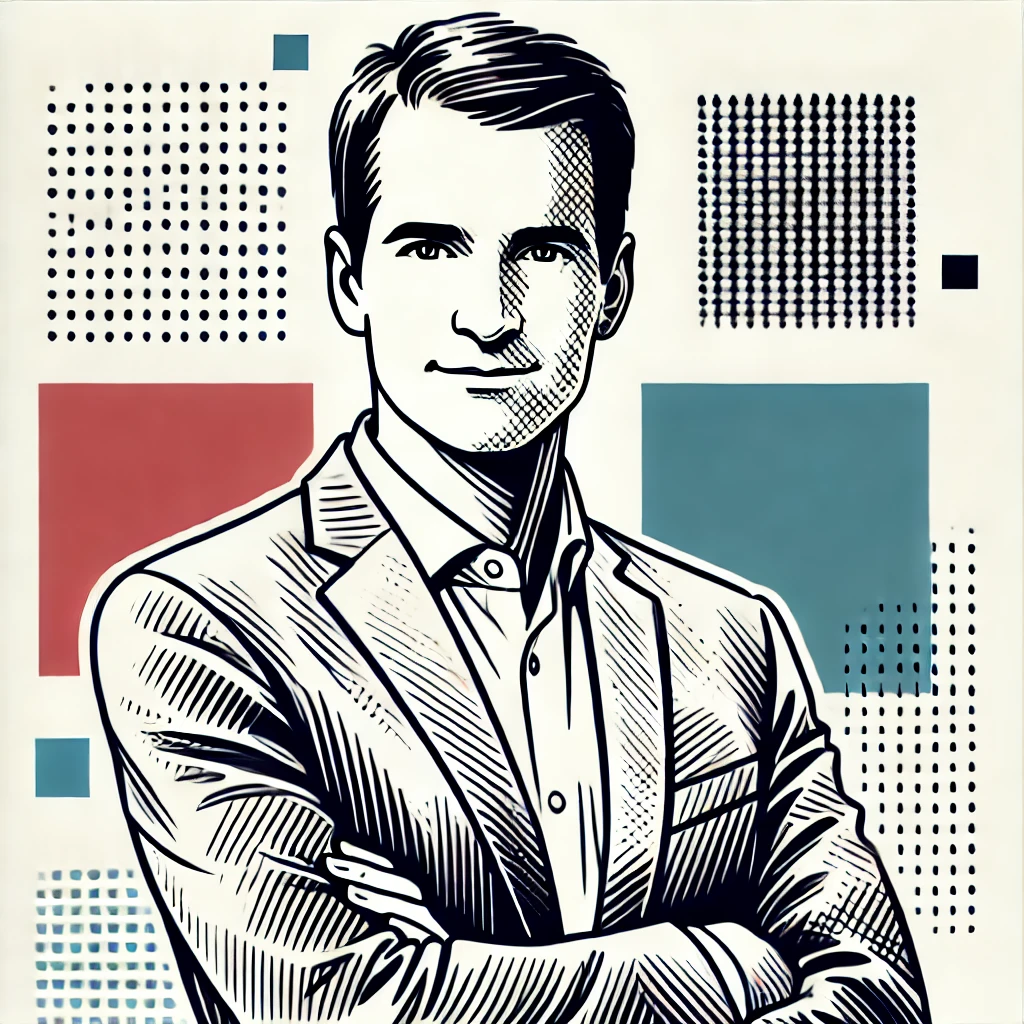
We know that LLM based chat bots can level up low-performers to the same standard as high-performers. But how AI can help high performers is less clear, partly because high-performers seem to need more convincing to use AI tools.
One study looked at doctors and their diagnostic accuracy with and without the help of AI. One group were asked to diagnose a set of cases on their own, a second set of doctors had access to GPT-4 to help their diagnoses. The first group scored correctly diagnosed 73% of cases. The second, 77%.
But GPT-4, given the same set of cases to diagnose on its own, scored 88% accuracy.
One interpretation of these results is that the doctors using GPT-4 sometimes disagreed with the AI's correct diagnosis and overruled it. Another is that they didn't bother to use it at all.
Behind both of those behaviours is a lack of trust in the AI. The doctors haven't seen any evidence that GPT-4 is a better diagnostician than they are, so they have no reason to believe that it might be right when it disagrees with them.
I really loved an idea from Julie Zhou on the three ingredients for trust: shared intent, the right skills, necessary context. She's talking about trust between humans, but it absolutely counts for human-AI collaboration too. The doctors may assume shared intent but they might find it harder to believe the AI has the right skills. It hasn't been to medical school. It hasn't completed its residency. How can we expect a doctor, whose every colleague has been educated in the same system, to believe that a general purpose chat bot who predicts the next most likely word in a sentence, could have the right skills? As for necessary context, in this case the AI only knows what the doctor tells it. While the doctor may give it the information they think it needs to know, when they get a different answer back, they may reason that their intuition is using some other piece of information the AI doesn't have access to. To me, there is an element of professional tribalism at play here too. The chatbot is, fundamentally, not a doctor so its opinion is not valid.
It's worth mentioning that the three ingredients above build cognitive trust. But there is another form of trust too. Affective trust is the belief that another person will act in your best interests and is based on your feelings about the person. Studies in financial decision making suggest that we are less likely to use AI in high stakes decisions because we need that affective trust, something that is easier to develop with humans and, tribalism again, something that we develop more easily with people who are similar to us. (However, chat bots like Pi are designed to be empathetic, leading to the development of affective trust. That would be an interesting future research direction.)
Another thing driving these behaviours is professional identity. This is one place the literature on AI adoption and real world experience doesn't seem to add up. I see and hear lots of people using AI for work every day to a far greater extent than it would appear in the literature. Putting to one side my clear sampling bias, I believe this is because people are using AI for aspects of their work that don't threaten their professional identity.
Imagine the study had been extended to include copywriters alongside the doctors and, as well as diagnosing a set of case studies, everyone had to write an effective sales email. The doctor would reason, 'Of course AI can help me write a sales email, that's not my area of expertise. But it can't help me diagnose this case study, I'm the expert there.' While the copywriter thinks, 'Of course AI can't help me write a sales email, that's my expertise. But it can definitely help me diagnose these case studies.'
This is a weird form of illusory superiority (you know, the cognitive bias where everyone thinks they're an above average driver). But it's also perfectly rational when, like those doctors, you have spent many years building up your expertise in a subject and you have to ask yourself what becomes of you if a machine can do your job? (Digression: I know of one role where workers were replaced by trained dolphins. Being ousted by different forms of intelligence isn't a new thing.)
Professional identity also trips us up when we hold a fixed view of the role. This is the 'real man' problem. (I've just made that up. But you know I'm driving at the Viking story of the boy who got lost in the woods and endured a freezing night with a pillow made of snow. When his father found him he kicked away the snow and shouted, "Real men don't use pillows." You knew that was the story I meant, right?) It's the rejection of a tool because it doesn't fit in with our view of how 'real' professionals operate. Real doctors don't need AI to help them diagnose cases. Real judges don't need AI to help them determine sentencing. Real taxi drivers don't need AI to help them find the best route. Others in the profession look down on people who 'need' these tools and that makes people reject them for fear of losing the respect of their peers.
So how can we help people adopt AI tools for the things they're already good at? Here's three things you can do:
- Make their use acceptable. This might be the hardest given many companies still have bans in place on general purpose LLM based AI. But you need to champion the positive outcomes that come from their use. Show people they won't be penalised for using them, that it won't impact their professional standing.
- Design for trust. This might be tricky if you can't develop your own software, but building affective trust into AI tools, making them feel empathetic, should make them more likely to be used. If you can't build it, look for off the shelf tools that can do this.
- Reframe the relationship between human and AI. Efficiency might be the business case for AI in organisations, but it is often not the goal of the person using the tool. When I hear positive stories about the use of AI they frame a chatbot as a sparring partner, a challenger, a critical listener. These are roles where critique is acceptable.
There is something different about using generative AI tools in decision making compared to previous waves of software advances. I think part of it is that it can feel like there is another mind at work, rather than the retrieval of information. While chatbots remain the interface between humans and AI, we should introduce them to teams not in the way we would rollout a tool, but in the way we would onboard a new colleague.